The enthusiasm for artificial intelligence (AI) across C-suites and boardrooms has ignited both excitement and pressure for rapid adoption. From streamlining decision-making and automating processes to unlocking insights from vast datasets, AI promises to propel businesses toward greater efficiency, innovation, and growth. However, successful AI adoption requires more than enthusiasm or financial investment. Achieving meaningful, reliable AI outcomes depends on the readiness of the foundational elements within an organization.
If you’re asking, “Is my business AI-ready?” the following key areas provide a roadmap to assess and enhance your organization’s AI preparedness, positioning it to harness AI with purpose and reliability.
Data Quality
High-quality data is essential to powering effective AI models. According to a Harvard Business Review study, 87% of respondents indicate that their data lacks the necessary accuracy for AI models to provide reliable reporting and predictions, and 80% cite inconsistent data formats as a significant obstacle. Poor-quality data introduces noise and errors, leading to unreliable AI outputs, wasted resources, and flawed decision-making.
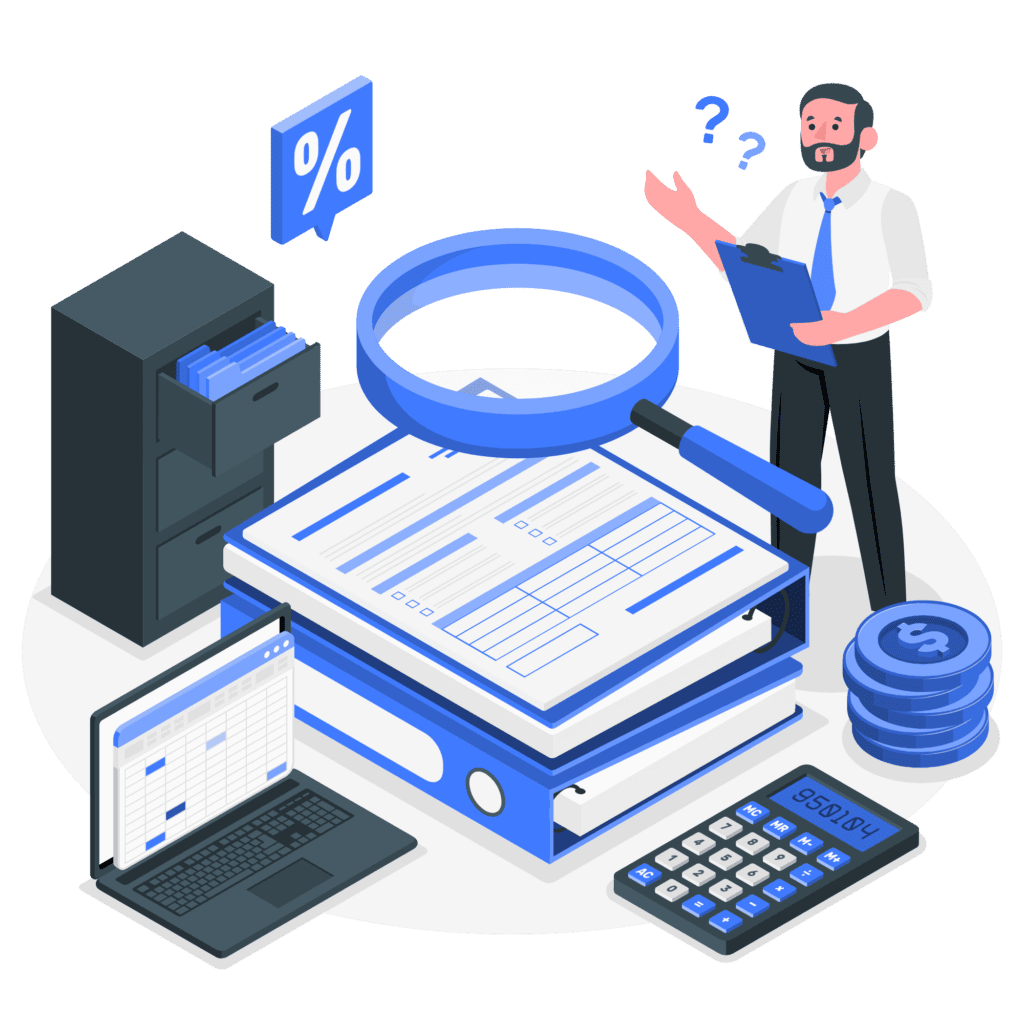
As Andrew Ng, Professor of AI at Stanford University and founder of DeepLearning.AI, emphasized, “If 80 percent of our work is data preparation, then ensuring data quality is the most critical task for a machine learning team.”
To produce meaningful results, AI models require data that is not only accurate but also representative of the problem being solved. Issues like missing values, duplicates, and inconsistent formats can disrupt AI training and affect predictive accuracy, often resulting in underfitting or overfitting.
- Steps to Assess: Conduct regular data profiling and auditing to identify gaps such as missing values, duplicates, and inconsistencies. Use these audits to pinpoint quality issues, then implement robust data-cleansing processes to standardize formats and remove errors.
- Key Practices: Establish data validation rules and quality metrics and introduce continuous data quality monitoring as part of your governance strategy. This approach ensures data remains reliable over time, allowing AI models to evolve and improve as new data is introduced.
Data Governance Framework
Data governance is the framework that ensures data consistency, security, and traceability—key to responsible AI adoption. A strong data governance framework provides clarity on data ownership, access rights, and usage policies, establishing the ethical and legal foundation for AI applications.
- Steps to Assess: Define clear data ownership, access permissions, and usage policies, and ensure compliance with relevant regulations. Establish protocols for ethical AI practices, covering both data and decision-making transparency.
- Key Practices: Appoint data stewards to oversee data governance, ensuring compliance with privacy and security standards, such as GDPR or CCPA. Implement privacy-by-design principles and regular audits to maintain trust and safeguard sensitive information, laying the groundwork for sustainable AI initiatives.
Beyond data management, governance includes establishing AI-specific policies on data usage and decision-making roles, helping companies mitigate unintended consequences and adhere to regulatory standards. For example, governance protocols define what data is accessible to AI, who can use AI, and under what conditions, ensuring responsible and compliant AI use.
Data Volume Management
AI’s transformative potential comes with a significant challenge: managing the massive volumes of data AI generates and requires for effective processing. Without a clear data volume management (DVM) strategy, businesses face spiraling storage costs, reduced system performance, and compliance risks.
- Key Practices: Conduct a comprehensive volume assessment to evaluate the current situation. Implement DVM tools, like Data ASSIST, to optimize storage costs and enhance system performance. Adopt retention management as part of your ILM strategy to align with data privacy regulations and improve data governance.
For businesses entering the AI space, managing the data deluge is non-negotiable. Structured and unstructured data must be handled cohesively to meet AI’s intensive data demands.
Data Accessibility
For true AI readiness, businesses need seamless access to large, diverse, and high-quality datasets. However, data accessibility goes beyond availability, but it is often facilitated by centralized sources of truth. Without these, an organization’s AI initiatives may be hindered by data silos or rest, resulting in missed opportunities and potential security risks.
- Steps to Assess: Evaluate whether data is fragmented across multiple systems or centrally accessible. Assess if data is stored in a manner that supports fast retrieval, especially for real-time applications, essential for applications such as fraud detection, recommendation engines, and predictive analytics.
Data Integration and Interoperability
A staggering 87% of companies cite data silos as a major challenge in AI adoption. Organizations often operate with multiple applications and environments that lack connectivity, which limits AI’s potential to work effectively across all data sources. For AI to produce reliable, unified insights, data must be consolidated from disparate systems into a single source of truth.
- Key Practices: Data integration and interoperability involve consolidating information across databases, ERP systems, and third-party applications to ensure that AI has access to a cohesive, accurate dataset.
Research points to the inability to plan for and pivot legacy systems as the second biggest barrier of entry for AI adoption. Having a comprehensive plan for your legacy systems, including a legacy retirement or decommissioning tool that consolidates the information into a single source.
Unstructured Data Management
Unstructured data accounts for 80% to 90% of the data generated and stored globally today. Unlike structured data, which fits neatly into rows and columns, unstructured data includes emails, videos, social media posts, and documents. While inherently complex, unstructured data provides invaluable context and insights when harnessed effectively.
Centralizing unstructured data into a unified content management platform, such as OpenText Extended ECM for SAP, is a game-changer. These platforms link unstructured data with business processes, enabling AI to access relevant content with relevant context information.
Unified repositories streamline the ingestion process and enhance data governance, ensuring data integrity, compliance, and controlled access. Moreover, the vast volumes of unstructured data can support AI models requiring extensive datasets for training and inference, making it a valuable asset for advancing AI capabilities.
Data Security and Privacy
Data security and privacy are paramount for organizations adopting AI, especially as these systems often process sensitive or proprietary information. According to KPMG’s AI survey, cybersecurity remains a top priority among organizations considering AI adoption.
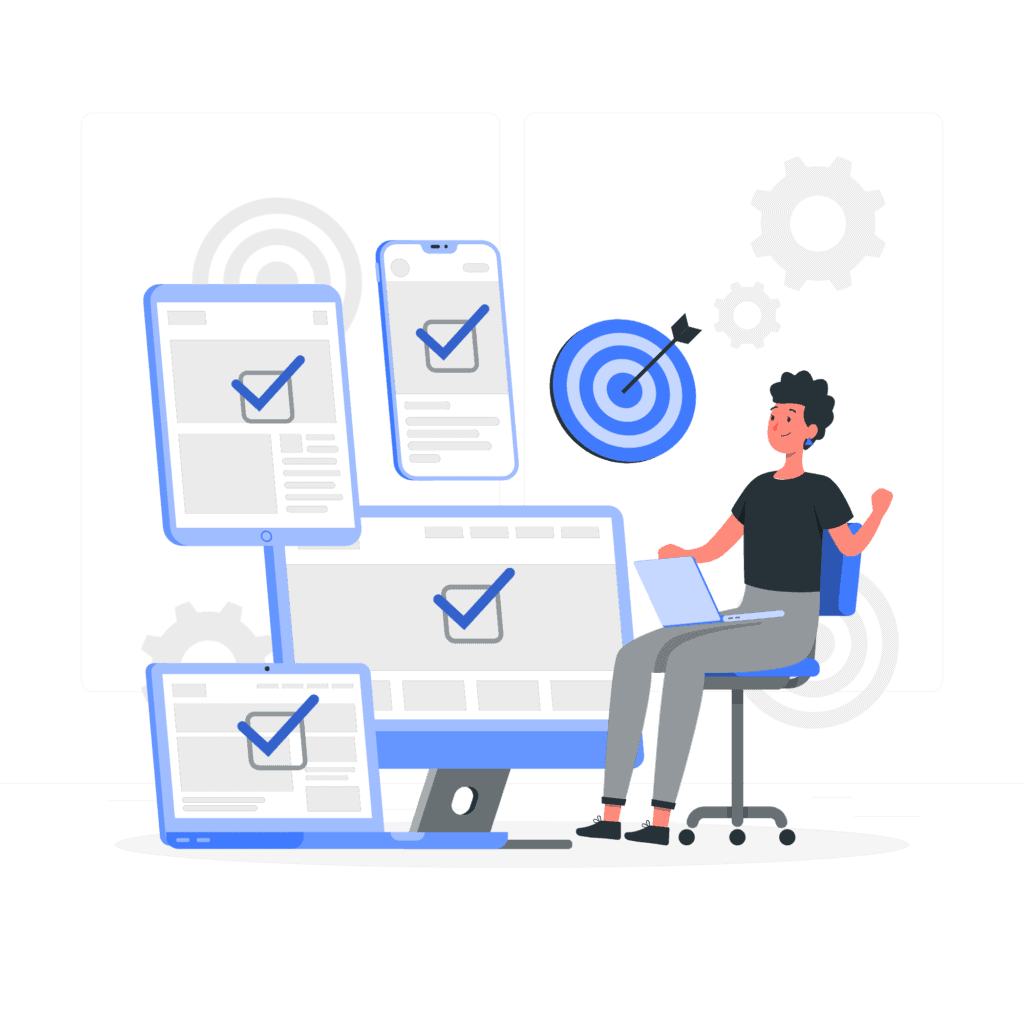
With increased regulatory oversight around personal data (e.g., GDPR, CCPA), robust security measures are non-negotiable. Data security and privacy controls protect both the data and the organization’s reputation, mitigating risks associated with data breaches and regulatory non-compliance.
- Steps to Assess: Check for encryption protocols, data access policies, and anonymization techniques to ensure data is protected throughout its lifecycle. Confirm compliance with industry regulations, especially if personal data is involved.
- Key Practices: Implement role-based access controls, conduct regular security audits, use encryption for data both at rest and in transit, and apply anonymization to safeguard personal information. These practices create a secure environment where AI models can access and use data confidently, reducing the risks of data leakage or misuse.
Strategic Alignment
Overall, a common misstep is deploying AI without clear alignment to strategic business goals, which can drain resources and miss valuable opportunities. An AI-ready organization begins by identifying specific objectives—whether enhancing customer experience or reducing operational costs—and then develops AI use cases that support these aims.
Deepak Sood, CEO at Auritas, explains that “focusing on the allure of “shiny objects” without a clear understanding of how they contribute to your goal usually leads to misguided efforts and wasted resources.” By evaluating each AI initiative for strategic relevance, businesses can ensure that their AI efforts drive meaningful and sustainable value.
By prioritizing these foundational data aspects, organizations can circumvent common challenges and lay the groundwork for truly impactful AI initiatives. Preparing with a solid AI-ready framework positions organizations to adopt AI successfully.
Explore how customers are thriving with Auritas innovation services and solutions.